Being able to prevent critical failures before they occur means greater network resilience—something that will become increasingly important as an ever-growing number of devices connect to networks.
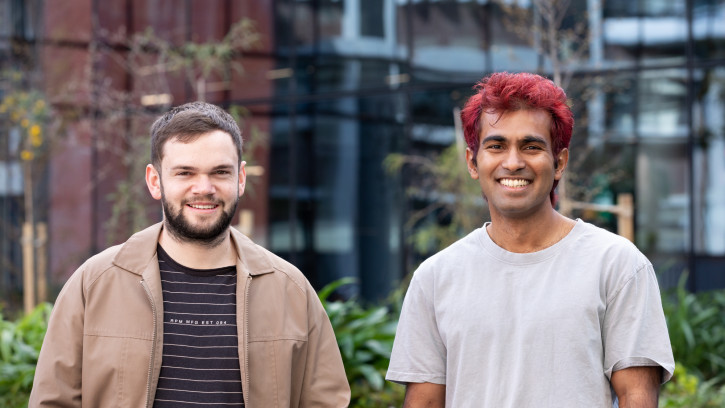
The entrepreneurs: Duncan Cameron and Murugaraj (Muru) Odiathevar.
Network reliability is essential to a wide range of industries such as the energy sector, telecommunications, environmental monitoring, financial services and many others. With more and more users accessing networks—and the Internet of Things (IOT) bringing sensor usage to new levels—network data flows have never been higher.
Being able to trust that data is, therefore, fundamental to the reliable operation of a network; the consequences of network failure can cause serious financial impact to both its operators and users.
Traditional network monitoring, however, is a slow, manual, expensive process that predominantly uses threshold analysis to score and characterise data—making it difficult to anticipate a problem or identify the underlying cause.
Two Victoria University of Wellington students (one current PhD student and one recent Master’s graduate) and their supervisors may have the answer—with a game-changing, automated machine-learning protocol that could help to predict physical or digital network failures before they actually happen.
The protocol uses machine-learning algorithms to process thousands of data points almost infinitely faster than any human being could—essentially teaching machines to adapt and respond over time to evolving network issues in a way that’s never been done before. The novel methodology not only allows data to be accurately and easily characterised—i.e. is the data normal (expected) or abnormal (a potential fault)—but also enables network operators to trust that characterisation.
Wellington UniVentures is working with the team to help turn their idea into a product that could ultimately change the way network operators monitor and maintain their networks.
Features and benefits
Increased network resilience
Reduced costs
By reducing network downtime, network operators save both time and money.
Future-proof
As networks continually evolve, it becomes difficult for human operators to continually keep up-to-date with the latest anomalies. The unique, automated algorithm enables networks to continually identify new anomalies and predict outages.
Next steps
Having conducted trials in a regional broadband provider’s network, the team is now looking to connect and partner with other network operators across a broad range of industries—for example, electrical distribution boards, telecommunications and data analysis companies—for a second round of trials to ensure they understand a broader range of industry needs before taking it to market.
At the end of the field trials, the team is aiming to have a service that could be applied to a wide range of industries with the goal of improving their current network monitoring to allow for better service optimisation.
Wellington UniVentures’ partner KiwiNet not only provided funding to develop the first prototype, the organisation also welcomed Murugaraj (Muru) Odiathevar and his fellow team member Duncan Cameron, into its Emerging Innovator Programme. This represents the first time a team—rather than an individual—has been invited into the programme.
For more information about this technology, please contact the Commercialisation Manager below.
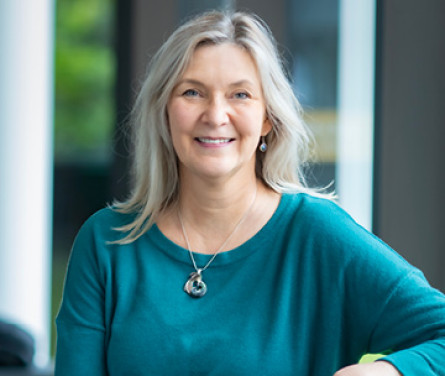